What is RFM analysis and why should I care?
Understanding your customers’ purchasing behaviors is crucial for effective marketing. One powerful tool that facilitates this understanding is RFM analysis, which stands for Recency, Frequency, and Monetary value. This method segments customers based on their purchasing patterns, enabling businesses to tailor their marketing strategies more precisely.
What are the three key factors?
- Recency: How recently a customer made a purchase.
- Frequency: How often a customer makes a purchase.
- Monetary: How much money a customer spends on purchases.
By scoring customers in each of these categories, businesses can identify their most valuable segments and predict future purchasing behaviors.
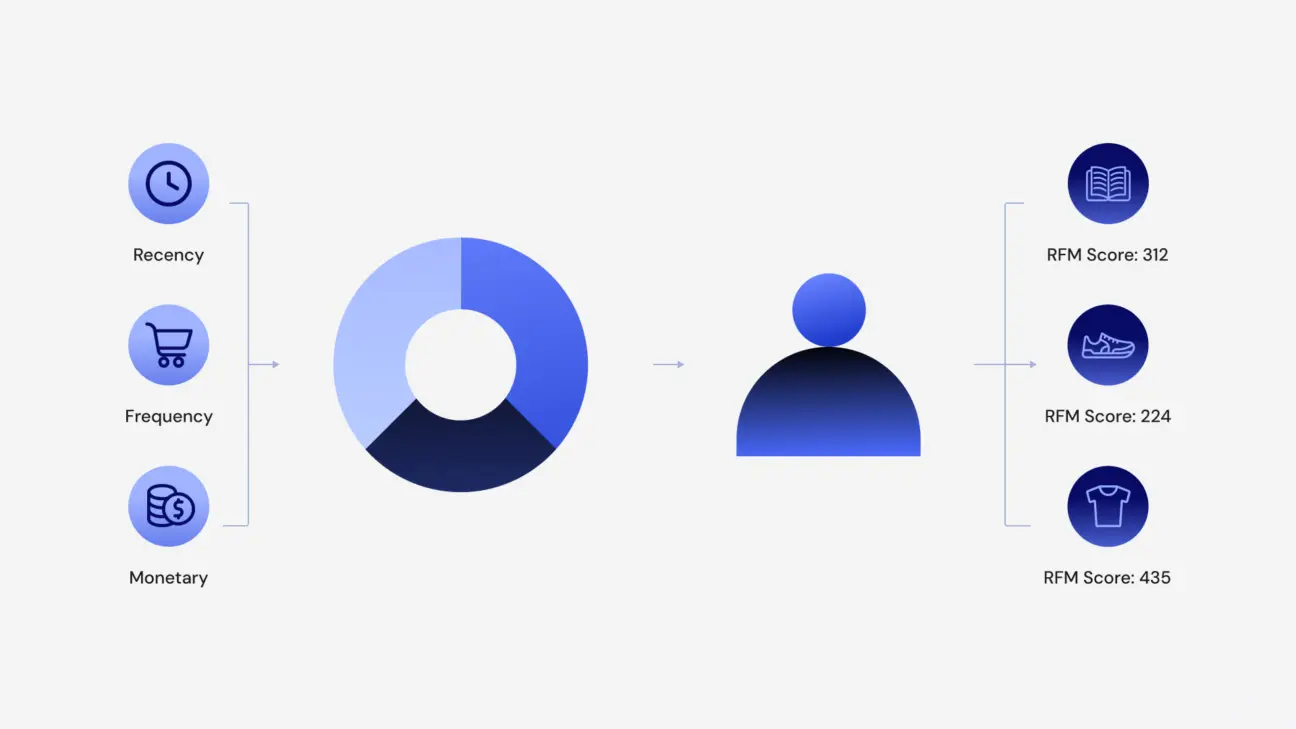
How to Conduct RFM Analysis
Data Collection
To get started with RFM analysis, gather relevant data on your customer's purchasing behaviour. This includes:
Recency: The date of the customer’s most recent purchase.
Frequency: How often the customer makes purchases within a specific timeframe (e.g., monthly, quarterly).
Monetary Value: The total amount spent by the customer over a period.
This data is typically available in your e-commerce platform, CRM system or POS software. Common tools you can use to collect and manage this data include Shopify, HubSpot and Salesforce.
Scoring
Once you’ve collected the data, it’s time to score customers based on the three RFM components. Typically, businesses assign a score between 1 and 5 for each factor (1 being the lowest and 5 the highest). Here’s how you can score customers:
Recency: Score customers based on how recently they made a purchase. Those who bought most recently receive a higher score.
Frequency: Score based on the number of purchases. Customers who buy more often get a higher score.
Monetary Value: Score based on the amount spent. High spenders get a higher score.
You can automate scoring using tools SegMetrics and Klaviyo.
Customer Segmentation
After scoring, group customers into segments. For example:
High Value Segment: Customers with high scores in all three categories (e.g., Recency 5, Frequency 5, Monetary 5).
At-Risk Segment: Customers who made a recent purchase but haven’t bought frequently or spent much (e.g., Recency 4, Frequency 1, Monetary 2).
Loyal Customers: Customers who buy regularly and spend moderately but aren’t recent buyers (e.g., Recency 3, Frequency 5, Monetary 4).
This segmentation allows you to target different customer groups with tailored strategies. Tools to help with segmentation include Mailchimp and Customer.io.
- Strategy Development
Once you've segmented your customers, develop specific marketing strategies for each segment. For example:
High Value Segment: Send them personalised offers, VIP rewards, or exclusive promotions to keep them engaged.
At-Risk Segment: Implement win-back campaigns or send re-engagement emails with discounts or reminders about their favourite products.
Loyal Customers: Offer loyalty rewards, referral bonuses, or other incentives to maintain their buying frequency.
To automate these strategies, you can use platforms like ActiveCampaign and Omnisend.
Benefits of RFM Analysis
There are 3 key advantages worth noting that RFM offers:
- Enhanced Customer Segmentation: Categorising customers based on RFM scores allows for more targeted marketing efforts.
- Improved Customer Retention: Identifying at-risk customers through declining recency or frequency scores enables timely interventions to re-engage them.
- Increased Revenue: Focusing on high-value customers can lead to more effective upselling and cross-selling opportunities.
Limitations and Considerations
While RFM analysis is a potent tool, it has its limitations. It may not be as effective for businesses where purchases are infrequent or for products with long sales cycles. Additionally, RFM doesn’t account for customer demographics or psychographics, which can also influence purchasing behaviour.
To overcome these limitations, integrating RFM analysis with other segmentation methods, such as cluster analysis or customer lifetime value models, can provide a more comprehensive understanding of customer behaviour. Leveraging machine learning algorithms can also enhance the precision of RFM segmentation, allowing for more personalized marketing efforts.